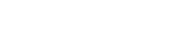
Dynamical systems with binary-valued observations are widely used in information industry, technology of biological pharmacy and other fields. Though there have been much efforts devoted to the identification of such systems, most of the previous investigations are based on first-order gradient algorithm which usually has much slower convergence rate than the Quasi-Newton algorithm. Moreover, persistence of excitation (PE) conditions are usually required to guarantee consistent parameter estimates in the existing literature, which are hard to be verified or guaranteed for feedback control systems. In this paper, we propose an online projected Quasi-Newton type algorithm for parameter estimation of stochastic regression models with binary-valued observations and varying thresholds. By using both the stochastic Lyapunov function and martingale estimation methods, we establish the strong consistency of the estimation algorithm and provide the convergence rate, under a signal condition which is considerably weaker than the traditional PE condition and coincides with the weakest possible excitation known for the classical least square algorithm of stochastic regression models. Convergence of adaptive predictors and their applications in adaptive control are also discussed.