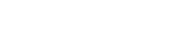
Machine learning has been successfully applied to various fields in computational science and engineering. In this paper, we propose interfaced neural networks (INNs) to solve interface problems with discontinuous coefficients as well as irregular interfaces. Unlike using a single network, which has been found to be almost unable to retain the inherent properties of interface problems, INN decomposes the computational domain into several subdomains according to the interface and leverages multiple networks, each of which is responsible for the solution on one subdomain. An extended multiple-gradient descent (MGD) method is introduced during the training phase, which utilizes multiple-gradient information to adaptively balance the interplay between different terms in the loss function. The effectiveness, accuracy and robustness of the proposed framework are demonstrated through a collection of interface problems in two and three spatial dimensions, including a moving interface case.
Publication:
Journal of Computational Physics, 2022, 10.1016/j.jcp.2022.111588.
Author:
Sidi Wu
State Key Laboratory of Scientific and Engineering Computing, National Center for Mathematics and Interdisciplinary Sciences, Academy of Mathematics and Systems Science, Chinese Academy of Sciences, Beijing 100190, China
School of Mathematical Sciences, University of Chinese Academy of Sciences, Beijing 100049, China
Benzhuo Lu
State Key Laboratory of Scientific and Engineering Computing, National Center for Mathematics and Interdisciplinary Sciences, Academy of Mathematics and Systems Science, Chinese Academy of Sciences, Beijing 100190, China
School of Mathematical Sciences, University of Chinese Academy of Sciences, Beijing 100049, China
Email: bzlu@lsec.cc.ac.cn
附件下载: