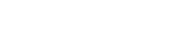
This study presents a high-accuracy, efficient, and physically induced method for 3D point cloud registration, which is the core of many important 3D vision problems. In contrast to existing physics-based methods that merely consider spatial point information and ignore surface geometry, we explore geometry aware rigid-body dynamics to regulate the particle (point) motion, which results in more precise and robust registration. Our proposed method consists of four major modules. First, we leverage the graph signal processing (GSP) framework to define a new signature, i.e., point response intensity for each point, by which we succeed in describing the local surface variation, resampling keypoints, and distinguishing different particles. Then, to address the shortcomings of current physics-based approaches that are sensitive to outliers, we accommodate the defined point response intensity to median absolute deviation (MAD) in robust statistics and adopt the X84 principle for adaptive outlier depression, ensuring a robust and stable registration. Subsequently, we propose a novel geometric invariant under rigid transformations to incorporate higher-order features of point clouds, which is further embedded for force modeling to guide the correspondence between pairwise scans credibly. Finally, we introduce an adaptive simulated annealing (ASA) method to search for the global optimum and substantially accelerate the registration process. We perform comprehensive experiments to evaluate the proposed method on various datasets captured from range scanners to LiDAR. Results demonstrate that our proposed method outperforms representative state-of-the-art approaches in terms of accuracy and is more suitable for registering large-scale point clouds. Furthermore, it is considerably faster and more robust than most competitors. Our implementation is publicly available at https://github.com/zikai1/GraphReg.
Publication:
IEEE Transactions on Image Processing, Volume 31, Pages: 7449 - 7464
https://doi.org/10.1109/TIP.2022.3223793
Author:
Mingyang Zhao
Beijing Academy of Artificial Intelligence, Beijing 100190, China
NLPR, Institute of Automation, CAS, Beijing 100190, China
Lei Ma
Beijing Academy of Artificial Intelligence, Beijing 100190, China
Institute for Artificial Intelligence, Beijing 100190, China
National Biomedical Imaging Center, Peking University, Beijing 100871, China
Xiaohong Jia
Academy of Mathematics and Systems Science, CAS, Beijing 100190, China
School of Mathematical Sciences, UCAS, Beijing 100149, China
Email: xhjia@amss.ac.cn
Dong-Ming Yan
NLPR, Institute of Automation, CAS, Beijing 100864, China
School of AI, UCAS, Beijing 101408, China
Tiejun Huang
National Engineering Research Center of Visual Technology, School of Computer Science
BAAI and Institute for Artificial Intelligence, Peking University, Beijing 100871, China
附件下载: