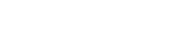
This paper considers the distributed sparse identification problem over wireless sensor networks such that all sensors cooperatively estimate the unknown sparse parameter vector of stochastic dynamic systems by using the local information from neighbors. A distributed sparse least squares algorithm is proposed by minimizing a local information criterion formulated as a linear combination of accumulative local estimation error and L_1-regularization term. The upper bounds of the estimation error and the regret of the adaptive predictor of the proposed algorithm are presented. Furthermore, by designing a suitable adaptive weighting coefficient based on the local observation data, the set convergence of zero elements with a finite number of observations is obtained under a cooperative non-persistent excitation condition. It is shown that the proposed distributed algorithm can work well in a cooperative way even though none of the individual sensors can fulfill the estimation task. Our theoretical results are obtained without relying on the independency assumptions of regression signals that have been commonly used in the existing literature. Thus, our results are expected to be applied to stochastic feedback systems. Finally, the numerical simulations are provided to demonstrate the effectiveness of our theoretical results.
Publication:
Automatica, Volume 151, May 2023, 110958
Authors:
Die Gan
Key Laboratory of Systems and Control, Institute of Systems Science, Academy of Mathematics and Systems Science, Chinese Academy of Sciences, Beijing 100190, PR China
School of Mathematical Sciences, University of Chinese Academy of Sciences, Beijing 100049, PR China
Zhongguancun Laboratory, Beijing 100094, PR China
Zhixin Liu
Key Laboratory of Systems and Control, Academy of Mathematics and Systems Science, Chinese Academy of Sciences, Beijing 100190, PR China
School of Mathematical Sciences, University of Chinese Academy of Sciences, Beijing 100049, PR China
Email: lzx@amss.ac.cn
附件下载: