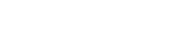
We consider the distributed optimization to minimize a sum of smooth and strongly convex local objective functions over directed graphs. Using row- and column-stochastic weights, we propose a family of distributed momentum methods. It is a parametric distributed momentum (PDM) method, for which different values of parameter can lead to different distributed momentum methods. PDM includes the distributed heavy ball method (ABm) and the distributed Nesterov gradient method (ABN) as its special cases. When the step sizes and the momentum coefficient do not exceed some upper bounds, we prove that PDM can converge to the optimal solution at a global R-linear rate. The convergence result of PDM not only covers that of ABm, but also supplements that of ABN which lacks theoretical convergence result. Simulation results on classification problems that arise in machine learning show that PDM with an appropriate negative parameter value can achieve faster acceleration than the existing distributed momentum algorithms.
Publication:
IEEE Transactions on Automatic Control PP(99):1-1
DOI:10.1109/TAC.2022.3160684
Author:
Juan Gao
School of Artificial Intelligence, Hebei University of Technology, Tianjin 300401, China
Xinwei Liu
Institute of Mathematics, Hebei University of Technology, Tianjin 300401, China
Yu-Hong Dai
LSEC, ICMSEC, Academy of Mathematics and Systems Science, Chinese Academy of Sciences, Beijing 100190, China
School of Mathematical Sciences, University of Chinese Academy of Sciences, Beijing 100049, China
Email: dyh@lsec.cc.ac.cn
Yakui Huang
Institute of Mathematics, Hebei University of Technology, Tianjin 300401, China
Peng Yang
State Key Laboratory of Reliability and Intelligence of Electrical Equipment and with the School of Artificial Intelligence, Hebei University of Technology, Tianjin 300401, China
附件下载: