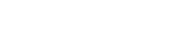
In the last decade, kernel-based regularization methods (KRMs) have been widely used for stable impulse response estimation in system identification. Its favorable performance over classic maximum likelihood/prediction error methods (ML/PEM) has been verified by extensive simulations. Recently, we noticed a surprising observation: for some data sets and kernels, no matter how the hyper-parameters are tuned, the regularized least square estimate cannot have higher model fit than the least square (LS) estimate, which implies that for such cases, the regularization cannot improve the LS estimate. Therefore, this paper focuses on how to understand this observation. To this purpose, we first introduce the squared error (SE) criterion, and the corresponding oracle hyper-parameter estimator in the sense of minimizing the SE criterion. Then we find the necessary and sufficient conditions under which the regularization cannot improve the LS estimate, and we show that the probability that this happens is greater than zero. The theoretical findings are demonstrated through numerical simulations, and simultaneously the anomalous simulation outcome wherein the probability is nearly zero is elucidated, and due to the ill-conditioned nature of either the kernel matrix, the Gram matrix, or both.
Publication:
Automatica, Volume 160, February 2024, 111442
http://dx.doi.org/10.1016/j.automatica.2023.111442
Author:
Biqiang Mu
Key Laboratory of Systems and Control, Academy of Mathematics and Systems Science, Chinese Academy of Sciences, Beijing 100190, China
Email: bqmu@amss.ac.cn
Lennart Ljung
Division of Automatic Control, Department of Electrical Engineering, Link?ping University, Link?ping 58183, Sweden
Tianshi Chen
School of Data Science and Shenzhen Research Institute of Big Data, The Chinese University of Hong Kong, Shenzhen 518172, China
附件下载: