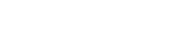
This article investigates adaptive identification and prediction problems for stochastic dynamical systems with saturated output observations, which arise from various fields in engineering and social systems, but up to now still lack comprehensive theoretical studies including guarantees for the estimation performance needed in practical applications. With this impetus, this article has made the following main contributions. First, to introduce an adaptive two-step quasi-Newton algorithm to improve the performance of the identification, which is applicable to a typical class of nonlinear stochastic systems with outputs observed under possibly varying saturation. Second, to establish the global convergence of both the parameter estimators and adaptive predictors, and to prove the asymptotic normality, under the weakest possible nonpersistent excitation condition, which can be applied to stochastic feedback systems with general nonstationary and correlated system signals or data. Third, to establish useful probabilistic estimation error bounds for any given finite length of data, using either martingale inequalities or Monte Carlo experiments. A numerical example is also provided to illustrate the performance of the proposed identification algorithm.
Publication:
IEEE Transactions on Automatic Control (Volume: 69, Issue: 3, March 2024)
https://doi.org/10.1109/TAC.2023.3314654
Key Laboratory of Systems and Control, Academy of Mathematics and Systems Science, Chinese Academy of Sciences, Beijing, China and School of Mathematical Science, University of Chinese Academy of Sciences, Beijing, China.
Key Laboratory of Systems and Control, Academy of Mathematics and Systems Science, Chinese Academy of Sciences, Beijing, China and School of Mathematical Science, University of Chinese Academy of Sciences, Beijing, China
Email: lguo@amss.ac.cn
附件下载: