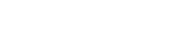
Regularized techniques, also named as kernel-based techniques, are the major advances in system identification in the last decade. Although many promising results have been achieved, their theoretical analysis is far from complete and there are still many key problems to be solved. One of them is the asymptotic theory, which is about convergence properties of the model estimators as the sample size goes to infinity. The existing related results for regularized system identification are about the almost sure convergence of various hyperparameter estimators. A common problem of those results is that they do not contain information on the factors that affect the convergence properties of those hyperparameter estimators, e.g., the regression matrix. In this article, we tackle problems of this kind for the regularized finite impulse response model estimation with the empirical Bayes (EB) hyperparameter estimator and filtered white noise input. In order to expose and find those factors, we study the convergence in distribution of the EB hyperparameter estimator, and the asymptotic distribution of its corresponding model estimator. For illustration, we run Monte Carlo simulations to show the efficacy of our obtained theoretical results.
Publication:
IEEE Transactions on Automatic Control (Volume: 68, Issue: 12, December 2023)
https://doi.org/10.1109/TAC.2023.3259977
Author:
School of Data Science and Shenzhen Research Institute of Big Data, The Chinese University of Hong Kong, Shenzhen, China
Key Laboratory of Systems and Control, Institute of Systems Science, Academy of Mathematics and System Science, Chinese Academy of Sciences, Beijing, China
Email: bqmu@amss.ac.cn
Division of Automatic Control, Department of Electrical Engineering, Linköping University, Linköping, Sweden
School of Data Science and Shenzhen Research Institute of Big Data, The Chinese University of Hong Kong, Shenzhen, China
附件下载: