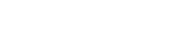
In this article, we develop a dynamical system counterpart to the term sparsity sum-of-squares algorithm proposed for static polynomial optimization. This allows for computational savings and improved scalability while preserving convergence guarantees when sum-of-squares methods are applied to problems from dynamical systems, including the problems of approximating region of attraction, the maximum positively invariant set, and the global attractor. At its core, the method exploits the algebraic structure of the data, thereby complementing existing methods that exploit causality relations among the states of the dynamical system. The procedure encompasses sign symmetries of the dynamical system as was already revealed for polynomial optimization. Numerical examples demonstrate the efficiency of the approach in the presence of this type of sparsity.
Publication:
IEEE Transactions on Automatic Control (Volume: 68, Issue: 12, December 2023)
https://doi.org/10.1109/TAC.2023.3293014
Author:
Academy of Mathematics and Systems Science, CAS, Beijing, China
Email: wangjie212@amss.ac.cn
Laboratory for Analysis and Architecture of Systems, CNRS, Toulouse, France
Laboratory for Analysis and Architecture of Systems, CNRS, Toulouse, France
Laboratory for Analysis and Architecture of Systems, CNRS, Toulouse, France
附件下载: