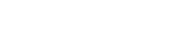
Imaging genetics integrates imaging and genetic techniques to examine how genetic variations influence the function and structure of organs like the brain or heart, providing insights into their impact on behavior and disease phenotypes. The use of organ-wide imaging endophenotypes has increasingly been used to identify potential genes associated with complex disorders. However, analyzing organ-wide imaging data alongside genetic data presents two significant challenges: high dimensionality and complex relationships. To address these challenges, we propose a novel, nonlinear inference framework designed to partially mitigate these issues.
Results
We propose a functional partial least squares through distance covariance (FPLS-DC) framework for efficient genome wide analyses of imaging phenotypes. It consists of two components. The first component utilizes the FPLS-derived base functions to reduce image dimensionality while screening genetic markers. The second component maximizes the distance correlation between genetic markers and projected imaging data, which is a linear combination of the FPLS-basis functions, using simulated annealing algorithm. In addition, we proposed an iterative FPLS-DC method based on FPLS-DC framework, which effectively overcomes the influence of inter-gene correlation on inference analysis. We efficiently approximate the null distribution of test statistics using a gamma approximation. Compared to existing methods, FPLS-DC offers computational and statistical efficiency for handling large-scale imaging genetics. In real-world applications, our method successfully detected genetic variants associated with the hippocampus, demonstrating its value as a statistical toolbox for imaging genetic studies.
Availability and implementation
The FPLS-DC method we propose opens up new research avenues and offers valuable insights for analyzing functional and high-dimensional data. In addition, it serves as a useful tool for scientific analysis in practical applications within the field of imaging genetics research. The R package FPLS-DC is available in Github: https://github.com/BIG-S2/FPLSDC.
Publication:
Bioinformatics (Volume: 40, Issue: 4, April 2024)
https://doi.org/10.1093/bioinformatics/btae173
Author:
Wenliang Pan
Academy of Mathematics and Systems Science, Chinese Academy of Sciences, Beijing, China
Email: panwliang@amss.ac.cn
Yue Shan
Departments of Biostatistics, Statistics, Genetics, and Computer Science and Biomedical Research Imaging Center, University of North Carolina, Chapel Hill, NC 27599, USA
Chuang Li
Department of Statistical Science, School of Mathematics, Sun Yat-sen University
, Guangzhou, China
Shuai Huang
Departments of Biostatistics, Statistics, Genetics, and Computer Science and Biomedical Research Imaging Center, University of North Carolina, Chapel Hill, NC 27599, USA
Tengfei Li
Departments of Radiology and Biomedical Research Imaging Center, University of North Carolina, Chapel Hill, NC 27599, USA
Yun Li
Departments of Biostatistics, Statistics, Genetics, and Computer Science and Biomedical Research Imaging Center, University of North Carolina, Chapel Hill, NC 27599, USA
Departments of Biostatistics, Statistics, Genetics, and Computer Science and Biomedical Research Imaging Center, University of North Carolina, Chapel Hill, NC 27599, USA
Departments of Radiology and Biomedical Research Imaging Center, University of North Carolina, Chapel Hill, NC 27599, USA
附件下载: