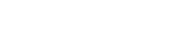
Spatial transcriptome technologies have enabled the measurement of gene expression while maintaining spatial location information for deciphering the spatial heterogeneity of biological tissues. However, they were heavily limited by the sparse spatial resolution and low data quality. To this end, we develop a spatial location-supervised auto-encoder generator STAGE for generating high-density spatial transcriptomics (ST). STAGE takes advantage of the customized supervised auto-encoder to learn continuous patterns of gene expression in space and generate high-resolution expressions for given spatial coordinates. STAGE can improve the low quality of spatial transcriptome data and smooth the generated manifold of gene expression through the de-noising function on the latent codes of the auto-encoder. Applications to four ST datasets, STAGE has shown better recovery performance for down-sampled data than existing methods, revealed significant tissue structure specificity, and enabled robust identification of spatially informative genes and patterns. In addition, STAGE can be extended to three-dimensional (3D) stacked ST data for generating gene expression at any position between consecutive sections for shaping high-density 3D ST configuration.
Publication:
Nucleic Acids Research (Volume: 52, Issue: 9, May 2024)
https://doi.org/10.1093/nar/gkae294
Author:
QShang Li
NCMIS, CEMS, RCSDS, Academy of Mathematics and Systems Science, Chinese Academy of Sciences, Beijing China; School of Mathematical Sciences, University of Chinese Academy of Sciences, Beijing, China
Kuo Gai
NCMIS, CEMS, RCSDS, Academy of Mathematics and Systems Science, Chinese Academy of Sciences, Beijing China; School of Mathematical Sciences, University of Chinese Academy of Sciences, Beijing, China
Kangning Dong
NCMIS, CEMS, RCSDS, Academy of Mathematics and Systems Science, Chinese Academy of Sciences, Beijing China; School of Mathematical Sciences, University of Chinese Academy of Sciences, Beijing, China
Yiyang Zhang
School of Software, Yunnan University, Kunming , China
Shihua Zhang
NCMIS, CEMS, RCSDS, Academy of Mathematics and Systems Science, Chinese Academy of Sciences, Beijing China; School of Mathematical Sciences, University of Chinese Academy of Sciences, Beijing, China; Key Laboratory of Systems Health Science of Zhejiang Province, School of Life Science, Hangzhou Institute for Advanced Study, University of Chinese Academy of Sciences, Chinese Academy of Sciences
Email: zsh@amss.ac.cn
附件下载: