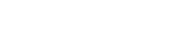
We study a class of polynomial optimization problems with a robust polynomial matrix inequality (PMI) constraint where the uncertainty set itself is also defined by a PMI. These can be viewed as matrix generalizations of semi-infinite polynomial programs because they involve actually infinitely many PMI constraints in general. Under certain sum-of-squares (SOS)-convexity assumptions, we construct a hierarchy of increasingly tight moment-SOS relaxations for solving such problems. Most of the nice features of the moment-SOS hierarchy for the usual polynomial optimization are extended to this more complicated setting. In particular, asymptotic convergence of the hierarchy is guaranteed, and finite convergence can be certified if some flat extension condition holds true. To extract global minimizers, we provide a linear algebra procedure for recovering a finitely atomic matrix-valued measure from truncated matrix-valued moments. As an application, we are able to solve the problem of minimizing the smallest eigenvalue of a polynomial matrix subject to a PMI constraint. If SOS convexity is replaced by convexity, we can still approximate the optimal value as closely as desired by solving a sequence of semidefinite programs and certify global optimality in case that certain flat extension conditions hold true. Finally, an extension to the nonconvexity setting is provided under a rank 1 condition. To obtain the abovementioned results, techniques from real algebraic geometry, matrix-valued measure theory, and convex optimization are employed.
Publication:
Mathematics of Operations Research, July 23, 2024
https://doi.org/10.1287/moor.2023.0361
Author:
Feng Guo
School of Mathematical Sciences, Dalian University of Technology, Dalian 116024, China;
Email: fguo@dlut.edu.cn
Jie Wang
Academy of Mathematics and Systems Science, Chinese Academy of Sciences, Beijing 100190, China
Email: wangjie212@amss.ac.cn
附件下载: