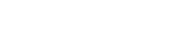
Given that gasoil plays a crucial role in carbon emission reduction, in this paper we propose a time-frequencybased interval decomposition ensemble (TFIDE) learning approach to forecast gasoil prices and capture the nonlinear impact of the global trend of low-carbon development on gasoil prices. The proposed method integrates bivariate empirical mode decomposition (BEMD), an interval multilayer perceptron (IMLP) network and a threshold autoregressive interval (TARI) model. First, we use BEMD to decompose interval-valued weekly gasoil prices into a finite number of complex-valued intrinsic mode function (IMF) components and a residual component. Second, we apply the IMLP model to forecast the IMFs and the TARI model to predict the residual part with predictors of carbon reduction technology and carbon emission concerns. After that, we combine all the forecasting results to generate the final gasoil price interval forecasting results. Our empirical results show that our carbon reduction technology variable improves middle-frequency IMF forecasting and that carbon emission concerns have a nonlinear impact on long-term gasoil price intervals. Furthermore, the proposed TFIDE approach outperforms other competing methods under different accuracy measurements.
Publication:
Energy Economics Volume 134, June 2024,
https://doi.org/10.1016/j.eneco.2024.107609
Author:
Zichun Yan
School of Economics and Management, Beijing University of Posts and Telecommunications, China
Fangzhu Tian
School of Economics and Management, Beijing University of Posts and Telecommunications, China
Yuying Sun
Academy of Mathematics and Systems Science, Chinese Academy of Sciences, China
School of Economics and Management and MOE Social Science Laboratory of Digital Economic Forecasts and Policy Simulation, University of Chinese Academy of Science, China
Email: sunyuying@amss.ac.cn
附件下载: