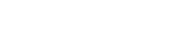
Kernel-based regularized system identification is one of the major advances in system identification in the past decade. A recent focus is to develop its asymptotic theory and it has been found that the Stein's unbiased risk estimator is asymptotically optimal (AO) in the sense of minimizing the mean squared error for prediction ability, but the empirical Bayes estimator is not AO in general. In this article, we further study the AO of various cross-validation (CV) estimators and show that the generalized CV method, leave k-out CV method, and r-fold CV method are all AO under mild assumptions, but the hold out CV method is not AO in general. We illustrate the efficacy of our theoretical results through numerical simulations.
Publication:
IEEE Transactions on Automatic Control ( Volume: 69, Issue: 7, July 2024)
http://dx.doi.org/10.1109/TAC.2023.3322576
Author:
Biqiang Mu
Key Laboratory of Systems and Control of CAS,
Academy of Mathematics and Systems Science, Chinese Academy of Sciences, Beijing 100190, China
Email: bqmu@amss.ac.cn
Tianshi Chen
School of Data Science, and Shen zhen Research Institute of Big Data, Chinese University of Hong Kong, Shenzhen 518172, China
Email: tschen@cuhk.edu.cn
附件下载: