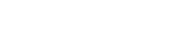
This paper focuses on multi-block optimization problems over transport polytopes, which underlie various applications including strongly correlated quantum physics and machine learning. Conventional block coordinate descent-type methods for the general multi-block problems store and operate on the matrix variables directly, resulting in formidable expenditure for large-scale settings. On the other hand, optimal transport problems, as a special case, have attracted extensive attention and numerical techniques that waive the use of the full matrices have recently emerged. However, it remains nontrivial to apply these techniques to the multi-block, possibly nonconvex problems with theoretical guarantees. In this work, we leverage the benefits of both sides and develop novel sampling-based block coordinate descent-type methods, which are equipped with either entropy regularization or Kullback Leibler divergence. Each iteration of these methods solves subproblems restricted on the sampled degrees of freedom. Consequently, they involve only sparse matrices, which amounts to considerable complexity reductions. We explicitly characterize the sampling-induced errors and establish convergence and asymptotic properties for the methods equipped with the entropy regularization. Numerical experiments on typical strongly correlated electron systems corroborate their superior scalability over the methods utilizing full matrices. The advantage also enables the first visualization of approximate optimal transport maps between electron positions in three-dimensional contexts.
Publication:
MATHEMATICS OF COMPUTATION published on August 15,2024
http://dx.doi.org/10.1090/mcom/3989
Author:
YUKUAN HU
State Key Laboratory of Scientific and Engineering Computing, Institute of Computational Mathematics and Scientific/Engineering Computing, Academy of Mathematics and Systems Science, Chinese Academy of Sciences, Beijing 100190, People’s Republicof China
University of Chinese Academy of Sciences, Beijing 100049, People’s Republic of China
Email: ykhu@lsec.cc.ac.cn
MENGYU LI
Institute of Statistics and Big Data, Renmin University of China, Beijing 100872, People’s Republic of China
Email: limengyu516@ruc.edu.cn
XIN LIU
State Key Laboratory of Scientific and Engineering Computing, Institute of Computational Mathematics and Scientific/Engineering Computing, Academy of Mathematics and Systems Science, Chinese Academy of Sciences, Beijing 100190, People’s Republic of China; and University of Chinese Academy of Sciences, Beijing 100049, People’s Republic of China
Email: liuxin@lsec.cc.ac.cn
CHENG MENG
Center for Applied Statistics, Institute of Statistics and Big Data, Renmin University of China, Beijing 100872, People’s Republic of China
Email: chengmeng@ruc.edu.cn
附件下载: