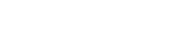
时间: 2023年11月2-3日
地点: 腾讯会议 73461626133
2023年11月2日 8:30-17:30
时 间 |
日 程 |
8:30-8:40 |
参会人员合影 |
8:40-9:20 |
报告人:席瑞斌(北京大学) 题 目:生物大数据的数学模型和方法 |
9:20-10:00 |
报告人:朱文圣(东北师范大学) 题 目:A Doubly Robust Estimation in Learning Optimal Individualized Treatment Regimes With Survival Outcomes |
10:00-10:40 |
报告人:侯 琳(清华大学) 题 目:Improving cross-ancestry genetic prediction with portable genetic effects |
10:40-11:20 |
报告人:王 涛(上海交通大学) 题 目:Analysis of sparse compositions of microbiomes |
11:20-12:00 |
报告人:潘小青(上海师范大学) 题 目:E-value: A superior alternative to P-value and its adjustments in DNA methylation studies |
|
|
13:30-14:10 |
报告人:刘耀午(西南财经大学) 题 目:A power-robust test for global hypotheses in generalized linear models |
14:10-14:50 |
报告人:邱宇谋(北京大学) 题 目:Information-incorporated Gene Network Construction with FDR Control |
14:50-15:30 |
报告人:刘 旭(上海财经大学) 题 目:Response best-subset selector for multivariate regression with large-scale response variables |
15:30-16:10 |
报告人:罗翔宇(中国人民大学) 题 目:Bayesian Integrative Region Segmentation in Spatially Resolved Transcriptomic Studies |
16:10-16:50 |
报告人:周 彦(深圳大学) 题 目:scDMV: A Zero-one Inflated Beta Mixture Model for DNA Methylation Variability with scBS-Seq Data |
16:50-17:30 |
报告人:赵世舜(吉林大学) 题 目:Risk Assessment of the Possible Intermediate Host Role of Pigs for Coronaviruses with a Deep Learning Predictor |
2023年11月3日 8:30-12:00
时间 |
日 程 |
8:00-8:40 |
报告人:张 洪(中国科技大学) 题 目:L0-regularized high-dimensional mediation analysis |
8:40-9:20 |
报告人:马 维(中国人民大学) 题 目:A New and Unified Family of Covariate Adaptive Randomization Procedures and Their Properties |
9:20-10:00 |
报告人:袁 敏(安徽医科大学) 题 目:Mutually exclusive spectral biclustering and its applications in cancer subtyping |
10:00-10:40 |
报告人:郭小波(中山大学) 题 目:儿童青少年近视预警模型的构建及其挑战 |
10:40-11:20 |
报告人:李正帮(华中师范大学) 题 目:An effective combination of the maximum and minimum Z-scores for testing sparse signals |
11:20-12:00 |
报告人:方红燕(安徽大学) 题 目:A greedy approach for mutual exclusivity analysis in cancer study |
报告1:生物大数据的数学模型和方法
报告人:席瑞斌(北京大学)
摘要:随着高通量组学技术的快速发展,生物医学研究已经进入了大数据时代。这些高通量组学技术特别是高通量测序技术已经在生物医学研究和临床实践中广泛应用,现代精准医学的发展也与这些高通量组学技术的发展密切相关。然而,这些新型生物医学数据的也对统计分析提出了巨大的挑战,在数据清洗、降维、去噪、数字特征提取、整合分析等方面都亟需发展新的统计方法。在本报告中,我将介绍我们最近发展的单细胞、空间转录组方法。
报告2:A Doubly Robust Estimation in Learning Optimal Individualized Treatment Regimes With Survival Outcomes
报告人:朱文圣
摘要: Precision medicine involves identifying optimal individual treatment regimes to extend survival time using survival data. Inverse probability weighting is a popular method used to estimate the value function of precision medicine. However, it is essential to consider both unbalanced covariates and the censor variable when dealing with survival data. Empirical evidence shows that the inverse probability weighting estimator is sensitive to slight misspecification of the models. To address this issue, we propose the contrast value function for survival data and provide an estimation for this function using derived optimal covariate balancing conditions. The estimator is doubly robust, that is, it is consistent when both the propensity score model and the censor probability model are correctly specified or when the outcome model is correctly specified. Additionally, asymptotic normality of the estimator can be established under standard regularity. Our method is demonstrated to be superior through numerous simulations, and we illustrate its effectiveness in the application of ACTG175 research and the GSE6532 cohort dataset.
报告3:Improving cross-ancestry genetic prediction with portable genetic effects
报告人:侯琳
摘要:Genome wide association analysis (GWAS) has provided numerous insights into the genetic etiology of complex diseases. Polygenic risk scores (PRS) calculated from genome-wide association studies (GWAS) of Europeans are known to have substantially reduced predictive accuracy in non-European populations, limiting their clinical utility and raising concerns about health disparities across ancestral populations. Here, we introduce a statistical framework named X-Wing to improve predictive performance in ancestrally diverse populations. X-Wing quantifies local genetic correlations for complex traits between populations, employs an annotation dependent estimation procedure to amplify correlated genetic effects between populations, and combines multiple population-specific PRS into a unified score with GWAS summary statistics alone as input. Through extensive benchmarking, we demonstrate that X-Wing pinpoints portable genetic effects and substantially improves PRS performance in non-European populations, showing 14.1%–119.1% relative gain in predictive R2 compared to state-of-the art methods based on GWAS summary statistics. Overall, X-Wing addresses critical limitations in existing approaches and may have broad applications in cross-population polygenic risk prediction.
报告4:Analysis of sparse compositions of microbiomes
报告人:王涛
摘要:A central objective in microbiome research is to identify microbes that play crucial roles in both health and disease, with the potential of these microbes to serve as biomarkers for preventing, diagnosing, and treating diseases. However, in microbiome studies, feature tables provide relative rather than absolute abundance of each feature in each sample, as the microbial loads of the samples and the ratios of sequencing depth to microbial load are both unknown and subject to considerable variation. Moreover, microbiome abundance data are count-valued, often over-dispersed, and contain a substantial proportion of zeros. The presence of compositionality, sparsity, and over-dispersion presents formidable challenges for absolute abundance analysis, leading to potentially misleading results when classical data analysis methods are applied. To address these challenges, we introduce a model-based approach called mbDecoda, for debiased analysis of sparse compositions of microbiomes. mbDecoda employs a zero-inflated negative binomial model, linking mean abundance to the variable of interest through a log link function, and it accommodates the adjustment for confounding factors. To efficiently obtain maximum likelihood estimates of model parameters, an Expectation Maximization algorithm is developed. A minimum coverage interval approach is then proposed to rectify compositional bias, enabling accurate and reliable absolute abundance analysis. Simulated examples and real-world data applications are used to comprehensively demonstrate the robustness and effectiveness of mbDecoda in the context of absolute abundance analysis.
报告5:E-value: A superior alternative to P-value and its adjustments in DNA methylation studies
报告人:潘小青
摘要: DNA methylation plays a crucial role in transcriptional regulation. Reduced representation bisulfite sequencing (RRBS) is a technique of increasing use for analyzing genome-wide methylation profiles. Many computational tools such as Metilene, MethylKit, BiSeq and DMRfinder have been developed to use RRBS data for the detection of the differentially methylated regions (DMRs) involved in epigenetic regulations of gene expression. For DMR detection tools, as for countless other medical applications, P-values and their adjustments are among the most standard reporting statistics used to assess the statistical significance of biological findings. However, P-values are coming under increasing criticism relating to their questionable accuracy and relatively high levels of false positive or negative indications. In this talk, I will introduce our method and R package ‘metevalue’ to calculate E-values, as likelihood ratios falling into the null hypothesis over the entire parameter space, for DMR detection in RRBS data. To evaluate the performance of E-values, we generated various RRBS benchmarking datasets using our simulator ‘RRBSsim’ with 8 samples in each experimental group. Our comprehensive benchmarking analyses showed that using E-values not only significantly improved accuracy, AUC and power, over that of P-values or adjusted P-values, but also reduced false discovery rates and type I errors. In applications using real RRBS data of CRL rats and a clinical trial on low-salt diet, the use of E-values detected biologically more relevant DMRs and also improved the negative association between DNA methylation and gene expression.
报告6:A power-robust test for global hypotheses in generalized linear models.
报告人:刘耀午
摘要:Testing a global hypothesis for a set of variables is a fundamental problem in statistics with a wide range of applications. A few well-known classical tests include the Hotelling's T2 test, likelihood ratio test, Wald test, and the empirical Bayes based score test. These classical tests, however, are not robust to the signal strength and could have a substantial loss of power when signals are weak or moderate, a situation we commonly encounter in contemporary applications. In this talk, I will introduce a Minimax Optimal Ridge-type Set Test (MORST), a simple and generic method for testing a global hypothesis. The power of MORST is robust and considerably higher than that of the classical tests when the strength of signals is weak or moderate. In the meantime, MORST only requires a slight increase in computation compared to these existing tests, making it applicable to the analysis of massive genome-wide data. We also provide the generalizations of MORST that are parallel to the traditional Wald test and Rao's score test in asymptotic settings. Extensive simulations demonstrated the robust power of MORST and that the type I error of MORST is well controlled. We applied MORST to the analysis of the whole-genome sequencing data from the Atherosclerosis Risk in Communities (ARIC) study, where MORST detected 20%--250% more signal regions than the classical tests.
报告7:Information-incorporated Gene Network Construction with FDR Control
报告人:邱宇谋
摘要:Large-scale gene expression studies allow gene network construction to uncover interactions among genes. To study direct interactions among genes, partial correlation-based networks are preferred over marginal correlations. However, FDR control for partial correlation-based network construction is not well-studied. In addition, currently available partial correlation-based methods cannot take existing biological knowledge to help network construction while controlling FDR. In this paper, we propose a method called Partial Correlation Graph with Information Incorporation (PCGII). PCGII estimates partial correlations between each pair of genes by regularized node-wise regression that can incorporate prior knowledge while controlling the effects of all other genes. It handles high-dimensional data where the number of genes can be much larger than the sample size and controls FDR at the same time. We compare PCGII with several existing approaches through extensive simulation studies and demonstrate that PCGII has better FDR control and higher power. We apply PCGII to a plant gene expression dataset where it recovers confirmed regulatory relationships and a hub node, as well as several direct interactions that shed light on potential functional relationships in the system.
报告8:Response best-subset selector for multivariate regression with large-scale response variables
报告人:刘旭
摘要:This article investigates the statistical problems of response variable selection with exponentially large-scale response variables for multivariate linear regression with two settings of fixed and diverging numbers of predictor variables. A response best-subset selection model is proposed by introducing a $0-1$ selection indictor to each response variable, and the response best-subset selector is developed, which is an efficient procedure for performing response variable selection and regression coefficient estimation simultaneously by introducing a separation parameter and a novel penalty function. The proposed response best-subset selectors for two settings both have consistency under mild conditions. For the fixed number of predictor variables, consistency and asymptotic normality are presented for the corresponding regression coefficient estimators. The Bonferroni test procedure with F-type test statistics turns out to be a special case of our response best-subset selector. In finite-sample simulation studies our response best-subset selector has stronger competitive advantages in keeping balance between higher accurate rates of important and unimportant response variables or larger Matthews correlation coefficient over its main competitors. A real data analysis demonstrates the effectiveness of the response best-subset selector for identifying dosage-sensitive genes.
报告9:Bayesian Integrative Region Segmentation in Spatially Resolved Transcriptomic Studies
报告人:罗翔宇
摘要:The spatially resolved transcriptomic study is a recently developed biological experiment that can measure gene expressions and retain spatial information simultaneously, opening a new avenue to characterize fine-grained tissue structures. We propose a nonparametric Bayesian method named BINRES to carry out the region segmentation for a tissue section by integrating all the three types of data generated during the study---gene expressions, spatial coordinates, and the histology image. BINRES is able to capture more subtle regions than existing statistical partitioning models that only partially make use of the three data modes and is more interpretable than neural-network-based region segmentation approaches. Specifically, due to a nonparametric spatial prior, BINRES does not require a prespecified region number and can learn it automatically. BINRES also combines the image and the gene expressions in the Bayesian consensus clustering framework and thus flexibly adjusts their contribution weights in a data-adaptive manner. A computationally scalable extension is developed for large-scale studies. Both simulation studies and the real application to three mouse spatial transcriptomic datasets demonstrate that BINRES outperforms the competing methods and easily achieves the uncertainty quantification of the integrative partition.
报告10:scDMV: A Zero-one Inflated Beta Mixture Model for DNA Methylation Variability with scBS-Seq Data
报告人:周彦
摘要:The whole genome bisulfite sequencing has been the gold standard of DNA methylation detection at single-nucleotide resolution on a genome-wide scale. Traditionally, sequencing methods can only get the average expression level of many cells and therefore ignore heterogeneity among individual cells. To observe the multilayered status of single cells, single-cell bisulfite sequencing (scBS-seq) technologies have been rapidly developed and proven to be an effective and powerful tool in identification of differentially methylated region (DMR). However, DMR recognition with scBS-seq has low precision accuracy since data are often sparse and have excess zeros and ones, due to the relatively low sequencing depth and low coverage. A new differential methylation analysis approach that can well accommodate the special features of such data and enhance recognition accuracy is most desirable. A new beta mixture approach (scDMV) that incorporates excess zeros and ones and allows low-input sequencing is proposed for single-cell bisulfite sequencing data to analyze methylation differences between samples from different groups for a site or region. Compared with several alternative methods, the scDMV approach performs favorably in terms of both sensitivity and precision and also has a good control of the false positive rate as shown in our extensive simulation studies. In real data applications, we also find that scDMV method exhibits higher precision and sensitivity in identifying differentially methylation regions, even for low-input samples. Furthermore, scDMV can delineate important information that is missed by other methods for GO enrichment analysis with single cell whole genome sequencing data. Availability: scDMV is available as an R package along with the tutorial at https://github.com/PLX-m/scDMV.
报告11:Risk Assessment of the Possible Intermediate Host Role of Pigs for Coronaviruses with a Deep Learning Predictor
报告人:赵世舜
摘要:Swine coronaviruses (CoVs) have been found to cause infection in humans, suggesting that Suiformes might be potential intermediate hosts in CoV transmission from their natural hosts to humans. The present study aims to establish convolutional neural network (CNN) models to predict host adaptation of swine CoVs. Decomposing of each ORF1ab and Spike sequence was performed with dinucleotide composition representation (DCR) and other traits. The relationship between CoVs from different adaptive hosts was analyzed by unsupervised learning, and CNN models based on DCR of ORF1ab and Spike were built to predict the host adaptation of swine CoVs. The rationality of the models was veri?ed with phylogenetic analysis. Unsupervised learning showed that there is a multiple host adaptation of different swine CoVs. According to the adaptation prediction of CNN models, swine acute diarrhea syndrome CoV (SADS-CoV) and porcine epidemic diarrhea virus (PEDV) are adapted to Chiroptera, swine transmissible gastroenteritis virus (TGEV) is adapted to Carnivora, porcine hemagglutinating encephalomyelitis (PHEV) might be adapted to Primate, Rodent, and Lagomorpha, and porcine deltacoronavirus (PDCoV) might be adapted to Chiroptera, Artiodactyla, and Carnivora. In summary, the DCR trait has been confirmed to be representative for the CoV genome, and the DCR-based deep learning model works well to assess the adaptation of swine CoVs to other mammals. Suiformes might be intermediate hosts for human CoVs and other mammalian CoVs. The present study provides a novel approach to assess the risk of adaptation and transmission to humans and other mammals of swine CoVs.
报告12:L0-regularized high-dimensional mediation analysis
报告人: 张洪
摘要:Mediation analysis can be used to investigate whether the causal effect of a predictor variable on an outcome variable is transmitted through mediators. In mediation analysis involving epigenetic biomarkers, the potential mediators are high-dimensional DNA methylation markers, which are crucial in the regulation of gene expression. There is a growing interest in developing statistical methods to test and estimate high-dimensional mediation effects utilizing various regularization techniques. However, the utilization of L0 regularization in high-dimensional mediation analysis remains unexplored. Furthermore, there is a lack of high-dimensional mediation methods in the context of Poisson regression models or accelerated failure time (AFT) models. In this work, we develop a novel L0-regularized high-dimensional mediation analysis (L0HMA) method. L0HMA can accommodate various types of outcomes in the context of linear regression, logistic regression, Poisson regression, proportional hazards and AFT models. Through comprehensive simulations, we demonstrate the superior performance of L0HMA in identification of mediators and estimation of mediation effects. Finally, we illustrate the application of L0HMA through two real DNA methylation datasets. Our proposed method has been implemented in the R package L0HMA available at https://github.com/zhaosaijun/L0HMA.
报告13:A New and Unified Family of Covariate Adaptive Randomization Procedures and Their Properties
报告人:马维
摘要:In clinical trials and other comparative studies, covariate balance is crucial for credible and efficient assessment of treatment effects. Covariate adaptive randomization (CAR) procedures are extensively used to reduce the likelihood of covariate imbalances occurring. In the literature, most studies have focused on balancing of discrete covariates. Applications of CAR with continuous covariates remain rare, especially when the interest goes beyond balancing only the first moment. In this talk, we propose a family of CAR procedures that can balance general covariate features, such as quadratic and interaction terms. Our framework not only unifies many existing methods, but also introduces a much broader class of new and useful CAR procedures. We show that the proposed procedures have superior balancing properties; in particular, the convergence rate of imbalance vectors is $O_P(n^{\epsilon})$ for any $\epsilon>0$ if all of the moments are finite for the covariate features, relative to $O_P(\sqrt n)$ under complete randomization, where $n$ is the sample size. Both the resulting convergence rate and its proof are novel. These favorable balancing properties lead to increased precision of treatment effect estimation in the presence of nonlinear covariate effects. The framework is applied to balance covariate means and covariance matrices simultaneously. Simulation and empirical studies demonstrate the excellent and robust performance of the proposed procedures.
报告14:Mutually exclusive spectral biclustering and its applications in cancer subtyping.
报告人:袁敏
摘要:Many soft biclustering algorithms have been developed and applied to various biological and biomedical data analyses. However, few mutually exclusive (hard) biclustering algorithms have been proposed to identify disease or molecular subtypes based on genomic or transcriptomic data. In this study, we developed a novel mutually exclusive spectral biclustering (MESBC) algorithm to detect mutually exclusive biclusters. MESBC simultaneously detects relevant features (genes) and corresponding patient subgroups and, therefore, automatically uses the signature features for each subtype to perform the clustering. Our simulations revealed that MESBC provided superior accuracy in detecting pre-specified biclusters compared with the non-negative matrix factorization (NMF) and Dhillon’s algorithm, particularly in very noisy data. Further analysis of the algorithm on real datasets obtained from the TCGA database showed that MESBC provided similar or more accurate overall survival prediction in patients with breast and lung cancer when compared to the existing, gold-standard subtypes for breast (PAM50) and lung cancer (integrative clustering). In the TCGA lung cancer patients, MESBC detected two clinically relevant, rare subtypes that were not detected by other biclustering or integrative clustering algorithms. Therefore, MESBC could potentially be used as a risk stratification tool to optimize the treatment for the patient, improve the selection of patients for clinical trials, and contribute to the development of novel therapeutic agents.
报告15:儿童青少年近视预警模型的构建及其挑战
报告人:郭小波
摘要: 近20年,全世界近视的发病率暴增,中国儿童青少年的近视问题引起了国家层面的高度关注。本报告首先基于国家最新发布的《儿童青少年近视防控公共卫生综合干预技术指南》,介绍国家对于近视防控的最新工作部署,并重点介绍近视预警管理方面的工作部署。接着,介绍近视预警模型研究领域最新研究进展。最后,介绍研究团队在近视预警研究领域开展的工作,并讨论该领域面临的挑战。
报告16:An effective combination of the maximum and minimum Z-scores for testing sparse signals
报告人:李正帮
摘要:In this paper, we propose a new method to combine these maximum and minimum Z-scores for testing sparse signals. Base on the new combination, we proposed a test that combines the maximum and minimum values from a large number of related Z-score values. We derive the asymptotic distribution of the new test under some conditions and null hypothesis. We investigate the theoretical power of our proposed test for some settings under alternative hypothesis. We also compare the power of our propose test to the existing test theoretically. Both extensive simulation results and real data analysis results show that our proposed test can control empirical type-1 error rates well and gain desirable powers. Our proposed method can be adopted easily and conveniently.
报告17:A greedy approach for mutual exclusivity analysis in cancer study
报告人: 方红燕
摘要:The main challenge in cancer genomics is to distinguish the driver genes from passenger or neutral genes. Cancer genomes exhibit extensive mutational heterogeneity that no two genomes contain exactly the same somatic mutations. Such mutual exclusivity (ME) of mutations has been observed in cancer data and is associated with functional pathways. Analysis of ME patterns may provide useful clues to driver genes or pathways and may suggest novel understandings of cancer progression. In this talk, we consider a probabilistic, generative model of ME, and propose a powerful and greedy algorithm to select the mutual exclusivity gene sets. The greedy method includes a pre-selection procedure and a stepwise forward algorithm which can significantly reduce computation time. Power calculations suggest that the new method is efficient and powerful for one ME set or multiple ME sets with overlapping genes. We illustrate this approach by analysis of the whole-exome sequencing data of cancer types from TCGA.
附件下载: