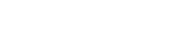
Most existing works on optimal filtering of linear time-invariant (LTI) stochastic systems with arbitrary unknown inputs assume perfect knowledge of the covariances of the noises in the filter design. This is impractical and raises the question of whether and under what conditions one can identify the process and measurement noise covariances (denoted as Q and R, respectively) of systems with unknown inputs. This article considers the identifiability of Q / R using the correlation-based measurement difference approach. More specifically, we establish 1) necessary conditions under which Q and R can be uniquely jointly identified; 2) necessary and sufficient conditions under which Q can be uniquely identified, when R is known; 3) necessary conditions under which R can be uniquely identified, when Q is known. It will also be shown that for achieving the results mentioned above, the measurement difference approach requires some decoupling conditions for constructing a stationary time series, which are proved to be sufficient for the well-known strong detectability requirements established by Hautus.
Publication:
IEEE Transactions on Automatic Control, Volume: 68, Issue: 7, July 2023
http://dx.doi.org/10.1109/TAC.2022.3208338
Author:
He Kong
Shenzhen Key Laboratory of Biomimetic Robotics and Intelligent Systems, Department of Mechanical and Energy Engineering, Southern University of Science and Technology, Shenzhen, China
Guangdong Provincial Key Laboratory of Human-Augmentation and Rehabilitation Robotics in Universities, Southern University of Science and Technology, Shenzhen, China
Salah Sukkarieh
Sydney Institute for Robotics and Intelligent Systems, The University of Sydney, Sydney, NSW, Australia
Travis J. Arnold
Tianshi Chen
School of Data Science and Shenzhen Research Institute of Big Data, The Chinese University of Hong Kong, Shenzhen, China
Biqiang Mu
Key Laboratory of Systems and Control, Institute of Systems Science, Academy of Mathematics and Systems Science, Chinese Academy of Sciences, Beijing, China
Email: bqmu@amss.ac.cn
Wei Xing Zheng
School of Computer, Data and Mathematical Sciences, Western Sydney University, Sydney, NSW, Australia
附件下载: