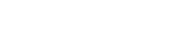
Physics-informed neural networks (PINNs) have emerged as an effective technique for solving PDEs in a wide range of domains. It is noticed, however, that the performance of PINNs can vary dramatically with different sampling procedures. For instance, a fixed set of (prior chosen) training points may fail to capture the effective solution region (especially for problems with singularities). To overcome this issue, we present in this work an adaptive strategy, termed failure-informed PINNs (FI-PINNs), which is inspired by the viewpoint of reliability analysis. The key idea is to define an effective failure probability based on the residual, and then, with the aim of placing more samples in the failure region, the FI-PINNs employs a failure-informed enrichment technique to adaptively add new collocation points to the training set, such that the numerical accuracy is dramatically improved. In short, similar to adaptive finite element methods, the proposed FI-PINNs adopt the failure probability as the posterior error indicator to generate new training points. We prove rigorous error bounds of FI-PINNs and illustrate their performance through several problems.
Publication:
SIAM Journal on Scientific ComputingVol. 45, Iss. 4 (2023)
http://dx.doi.org/10.1137/22M1527763
Author:
Zhiwei Gao
School of Mathematics, Southeast University, Nanjing 210096, China.
Liang Yan
School of Mathematics, Southeast University, Nanjing 210096, and Nanjing Center for Applied Mathematics, Nanjing 211135, China.
Tao Zhou
LSEC, Institute of Computational Mathematics, Academy of Mathematics and Systems Science, Chinese Academy of Sciences, Beijing 100190, China.
Email: tzhou@lsec.cc.ac.cn
附件下载: