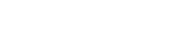
In this paper, we consider a class of nonconvex-nonconcave minimax problems, i.e., NC-PL minimax problems, whose objective functions satisfy the Polyak-Lojasiewicz (PL) condition with respect to the inner variable. We propose a zeroth-order alternating gradient descent ascent (ZO-AGDA) algorithm and a zeroth-order variance reduced alternating gradient descent ascent (ZO-VRAGDA) algorithm for solving NC-PL minimax problem under the deterministic and the stochastic setting, respectively. The total number of function value queries to obtain an $\epsilon$-stationary point of ZO-AGDA and ZO-VRAGDA algorithm for solving NC-PL minimax problem is upper bounded by $\mathcal{O}(\varepsilon^{-2})$ and $\mathcal{O}(\varepsilon^{-3})$, respectively. To the best of our knowledge, they are the first two zeroth-order algorithms with the iteration complexity gurantee for solving NC-PL minimax problems.
Publication:
Journal of Machine Learning Research 24 (2023) 1-25
https://jmlr.org/papers/volume24/22-1518/22-1518.pdf
Author:
Zi Xu
Department of Mathematics Shanghai University Shanghai 200444, People’s Republic of China
Newtouch Center for Mathematics of Shanghai University Shanghai University Shanghai 200444, People’s Republic of China
Zi-Qi Wang
Department of Mathematics Shanghai University Shanghai 200444, People’s Republic of China
Jun-Lin Wang
Department of Mathematics Shanghai University Shanghai 200444, People’s Republic of China
Yu-Hong Dai
LSEC, ICMSEC, Academy of Mathematics and Systems Science, Chinese Academy of Sciences Academy of Mathematics and Systems Science, Chinese Academy of Sciences Beijing 100190, China
Email: dyh@lsec.cc.ac.cn