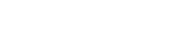
The leaky ReLU network with a group sparse regularization term has been widely used in the recent years. However, training such network yields a nonsmooth nonconvex optimization problem and there exists a lack of approaches to compute a stationary point deterministically. In this paper, we first resolve the multi-layer composite term in the original optimization problem by introducing auxiliary variables and additional constraints. We show the new model has a nonempty and bounded solution set and its feasible set satisfies the Mangasarian-Fromovitz constraint qualification. Moreover, we show the relationship between the new model and the original problem. Remarkably, we propose an inexact augmented Lagrangian algorithm for solving the new model, and show the convergence of the algorithm to a KKT point. Numerical experiments demonstrate that our algorithm is more efficient for training sparse leaky ReLU neural networks than some well-known algorithms.
Publication:
Journal of Machine Learning Research, 2023, Volume: 24, Issue: 212, Pages: 1?43
https://jmlr.org/papers/volume24/22-0491/22-0491.pdf
Author:
Wei Liu
Institute of Computational Mathematics and Scientific/Engineering Computing Academy of Mathematics and Systems Science Chinese Academy of Sciences Beijing 100190, China
Xin Liu
Institute of Computational Mathematics and Scientific/Engineering Computing Academy of Mathematics and Systems Science Chinese Academy of Sciences Beijing 100190, China
Email: liuxin@lsec.cc.ac.cn
Xiaojun Chen
Department of Applied Mathematics The Hong Kong Polytechnic University Hung Hom, Kowloon, Hong Kong
附件下载: