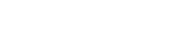
Consumer goods volume and their consumption structure significantly contribute to water pollution within economic zones. However, there remains a need to better comprehend the nuanced and seasonal nonlinear relationship between consumer goods and water pollution, which currently obstructs essential consumption adjustments aimed at reducing water pollution. This study selected an effective machine learning model Light Gradient Boosting Machine (LightGBM) with SHapley Additive Explanations (SHAP) to assess the nonlinear relationship between consumer goods and water pollution during flood and non-flood seasons within the Yangtze River Economic Belt from 2013 to 2022, Baidu Index of consumer goods were used to represent the potential consumption level (PCL). The findings revealed the following: (1) During flood seasons, hydroelectricity and the PCL of furniture and cultural goods emerged as factors driving water pollution reduction. Conversely, GDP, PCL of communication devices, and beverages played significant roles in exacerbating water pollution. During non-flood seasons, the drivers for reducing water pollution included GDP, income gap and PCL of cultural goods. Meanwhile, PCL of cars, western medicine and oil became pivotal drivers for exacerbating water pollution. (2) Concerning consumption structure during flood and non-flood seasons, maintaining consumption levels of costume industrial goods, cultural, sports and health goods and transportation and accommodation goods below 66.71%, 77.66% and 79.61% respectively of their average PCL appeared beneficial in reducing water pollution. During flood seasons, consumption levels of cars and furniture below 85.69% and 91.46% respectively of their average PCL were associated with reduced water pollution. During non-flood seasons, consumption levels of cars and oil below 82.95% and 71.28% respectively of their average PCL could help reduce water pollution. (3) The proportion of hydroelectricity generation exceeding 2% and 3% respectively during flood and non-flood season.
Publication:
Journal of Cleaner Production (TOP, JCR Q1, IF=11.072), Vol.444, 141254 (2024).
https://doi.org/10.1016/j.jclepro.2024.141254
Authors:
Songhua Huan
Academy of Mathematics and Systems Science, Chinese Academy of Sciences, Beijing 100190, China
University of Chinese Academy of Sciences, Beijing 100049, China
Xiuli Liu (Corresponding author)
Academy of Mathematics and Systems Science, Chinese Academy of Sciences, Beijing 100190, China
University of Chinese Academy of Sciences, Beijing 100049, China
Center for Forecasting Science, Chinese Academy of Sciences, Beijing 100190, China
E-mail: xiuli.liu@amss.ac.cn
附件下载: